ORNL seismic research aims to mitigate risks in geothermal and carbon capture
Energy Disrupter
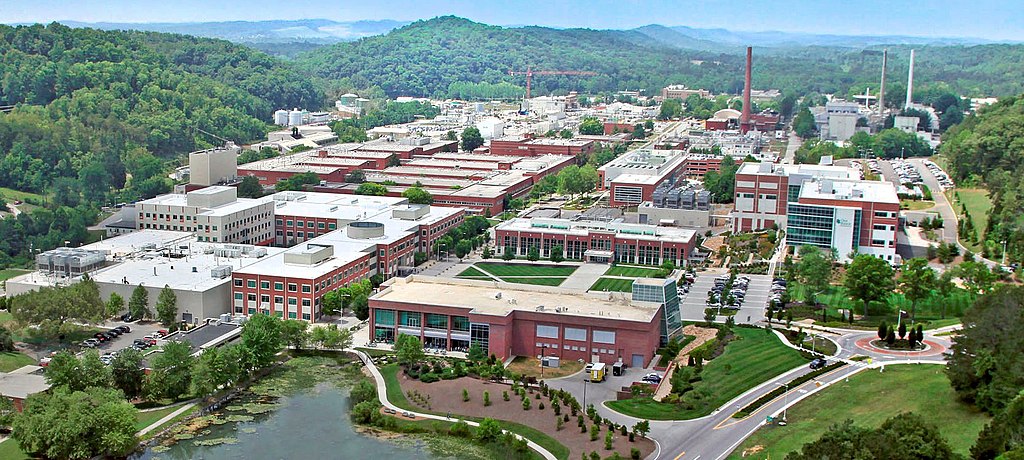
A research paper from ORNL explores the use of machine learning for faster, more accurate processing of seismicity data to help mitigate geothermal risks.
A research project by Chengping Chai, a geophysicist from the Department of Energy’s Oak Ridge National Laboratory, aims to develop faster and more accurate methods for the processing seismic data, thus reducing the risk of geothermal and carbon capture projects. This is done by leveraging machine learning techniques and advanced seismic data processing algorithms.
Chai also works closely with ORNL scientist Monica Maceira, who was already managing ORNL’s seismology portfolio when Chai was still a graduate student at Pennsylvania State University.
The goal of the project is to use seismology to better understand the location and movement of subsurface fractures, both for geothermal and carbon capture projects. Accurate seismic information can enhance both the safety and effectiveness of such ventures.
“We need to use these tools before, during and after these projects occur,” Chai said. “We need to understand the subsurface for choosing sites and drilling boreholes. During the pumping of water or carbon dioxide, we need to monitor how the fluid is moving and how the fracture is going. And afterward, we need to see if the fracture remains as intended or is evolving the way we want.”
Seismic monitoring with machine learning
The research paper “Machine Learning Enhanced Seismic Monitoring at 100 km and 10 m Scales” by Chai and Maceira can ba accessed via this link: https://www.osti.gov/biblio/1845768
The project involves the development of a machine learning-enhanced seismic monitoring workflow for the rapid and automatic processing of seismic event catalogs. The workflow combines cutting-edge machine learning techniques and advanced seismic data processing algorithms. It is suitable for the monitoring of both natural and induced seismicity at a wide range of spatial scales – from 10 meters to 100 kilometers.
Data was collected from two sites – the Oklahoma region for the 10-kilometer scale, and the EGS Collab site for the 10-meter scale.
For the Oklahoma scenario, around 235,000 three-component body wave seismograms were downloaded. Manual picking to measure the signal arrival times would have been very labor-intensive. The deep learning model speeds up this phase picking task, completing it in only 38 minutes compared to more than 100 days for a human analyst.
Deep learning also resulted in better seismic event locations. Tighter linear trends were observed for the deep-learning derived picks compared to the manual picks. The deep learning results also generally match with the moment tensor solutions from the Saint Louis University earthquake catalog.
Read the paper for a more detailed discussion of the project and its results.
Getting high-resolution information faster
According to Maceria, activities that involve extracting something from the subsurface or injecting something into the underground can cause a change in the state of stress, thus creating induced seismicity. This has been the case for Oklahoma, where an uptick in earthquakes coincided with the introduction of fracking.
A system that can process rapid, high-resolution seismic data can help operators maintain safety by allowing them to make on-the-spot decisions about altering operations. Geothermal projects can also benefit from accurate seismic models by helping to identify fractures that can act as permeable channels for fluids.
Chai is still refining the technique that was developed from the study, but the preliminary results from the deep learning approach show that a 99.9% reduction in processing time is possible.
Source: ORNL